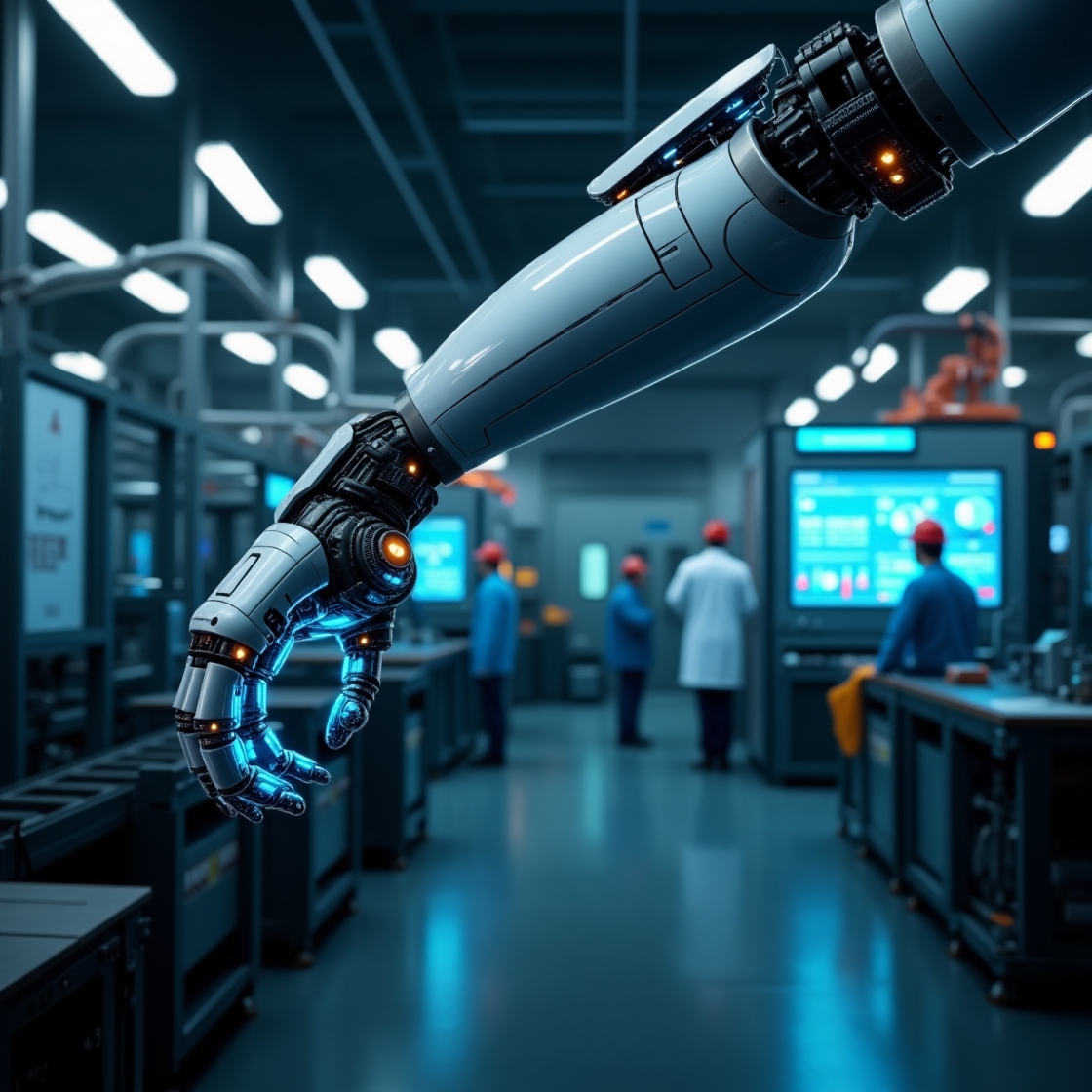
“Sir, everything is down! The customer portal, payment gateway, everything!”
Those words still echo in my mind from that fateful Monday morning. I was in the middle of our weekly leadership meeting at ValueDX headquarters in Pune when Rahul, our typically composed Head of IT Operations, burst through the door. The panic in his eyes told me this wasn’t a routine glitch.
Our entire digital infrastructure had crashed following a seemingly minor system update. For the next six hours, our team worked frantically to identify the root cause while our customer service team fielded hundreds of distressed calls. By the time we resolved the issue, we had lost an estimated ₹35 lakhs in business and immeasurable client goodwill.
That crisis became our turning point. As CTO of ValueDX, I knew we needed a fundamentally different approach to incident response. Our traditional methods simply weren’t keeping pace with our increasingly complex IT landscape. What followed was our journey into AI-powered incident management – a transformation that has not only dramatically reduced our downtime but revolutionized how our entire organization approaches operational resilience.
When Traditional Incident Response Falls Short
Before I share how AI changed our approach, let me paint a picture of the challenges we faced – challenges I’ve since discovered are painfully common across Indian enterprises.
During our system outage, our incident response looked something like this:
Rahul’s team received multiple alerts from different monitoring systems. Engineers scrambled to check various logs and dashboards. WhatsApp groups exploded with messages. Some team members were unreachable because they were commuting in Mumbai’s notorious traffic.
Meanwhile, our customer support center in Bengaluru was overwhelmed with calls, but had no clear updates to provide. Our sales team in Delhi was in the middle of a critical client presentation when they discovered the demo environment was down.
This chaotic scene reflects the fundamental limitations of traditional incident response:
- The time trap: Our engineers spent precious hours manually sifting through logs and alerts, trying to connect the dots while the business bled money.
- The signal-to-noise problem: Our monitoring systems generated over 200 alerts during the incident. Which ones mattered? Which were symptoms versus causes? Our team was drowning in data but starved for insights.
- The knowledge gap: Our senior DevOps engineer who had architected part of the affected system was on leave for his sister’s wedding in Jaipur. The team lacked his contextual knowledge, adding critical delays.
- The reactive cycle: We only knew about the problem after it had already impacted customers. By then, the damage was done.
A report by NASSCOM suggests Indian companies lose approximately ₹5 crore annually due to IT downtime on average. For larger enterprises, this figure can be substantially higher. In our increasingly digital economy, these losses are simply unsustainable.
Our AI Transformation Journey
Three weeks after our crisis, I found myself sharing a chai with Vivek, an old engineering college friend who now specialized in AIOps implementations. As I described our incident response challenges, he nodded knowingly.
“You’re fighting 21st-century complexity with 20th-century methods,” he observed. “It’s like trying to manage Bengaluru traffic with manual signals and traffic constables.”
That conversation sparked our AI incident response initiative. Here’s how it transformed our approach:
1. From Reactive to Predictive Detection
The first breakthrough came just two months after implementing our AI monitoring system. During Diwali season – our peak business period – the AI detected unusual patterns in our database response times. Though still within “normal” thresholds that wouldn’t have triggered traditional alerts, the system recognized these subtle anomalies as precursors to potential failure.
“The AI flagged that our payment database was showing early signs of memory issues,” Rahul told me, still sounding impressed months later. “We were able to implement fixes during scheduled maintenance instead of dealing with a crash during peak shopping hours.”
This shift from reactive to predictive detection has been transformative. Our AI continuously learns what “normal” looks like across thousands of metrics, identifying potential issues before they impact users or trigger traditional monitoring alerts.
2. Making Sense of Alert Storms
During another incident, a network configuration change triggered cascading issues across multiple systems. Traditional monitoring generated over 150 separate alerts.
“Previously, this would have overwhelmed us,” explained Priya, one of our senior SREs. “We’d spend the first critical hour just trying to determine which alerts mattered and how they were connected.”
Our AI correlation engine automatically grouped related alerts, suppressed redundant notifications, and visually mapped the relationship between symptoms and likely causes. What would have been an alert storm became an actionable diagnosis that guided our team directly to the misconfigured network settings.
“It was like having a senior architect instantly analyze the situation and guide our troubleshooting,” Priya noted. “The system directed us to the right place in minutes, not hours.”
3. Automated Resolution for Common Scenarios
Last quarter, we experienced a 3 AM incident when one of our application servers became unresponsive. Instead of waking an on-call engineer, our AI incident response system:
- Recognized the pattern from previous occurrences
- Executed the predefined playbook to restart the service and verify recovery
- Documented the incident with relevant logs and metrics
- Notified the team for review during working hours
“I checked my phone expecting the usual middle-of-the-night crisis,” Rahul recalled with a smile. “Instead, I found a message saying the issue had been automatically resolved three hours earlier. I actually got to sleep through the night for once.”
For standard incidents with established resolution paths, automation has eliminated the need for manual intervention entirely – reducing our mean time to resolution (MTTR) by 67% for common scenarios.
4. Empowering Teams with Contextual Knowledge
During a recent API gateway issue, one of our junior engineers was the first responder. In the past, this might have necessitated escalation, adding precious minutes or even hours to resolution time.
Instead, our AI system provided:
- Historical context about similar past incidents
- Links to relevant documentation and runbooks
- Specific diagnostic commands to run with expected outputs
- Recommendations based on successful past resolutions
“I was able to resolve an incident that previously would have been escalated to L3 support,” the engineer told me proudly during our monthly review. “The AI guided me through the troubleshooting steps as if our most experienced architect was looking over my shoulder.”
This knowledge democratization has been particularly valuable for our teams distributed across different cities and time zones, ensuring 24×7 high-quality incident response regardless of who is on call.
5. Learning and Improving with Every Incident
Perhaps the most powerful aspect of our AI incident response system is its ability to learn continuously. After each incident, the system analyzes:
- What detection methods worked or failed
- Which response actions were effective
- How similar scenarios might be prevented entirely
- Patterns that might predict future issues
“We’re not just responding faster – we’re having fewer incidents overall,” Rahul reported during our quarterly review. “The system identified that deployments made on Friday afternoons had a 38% higher failure rate, so we adjusted our release schedule accordingly.”
This continuous improvement loop has reduced our total incident volume by 43% over the past year, allowing our technical teams to focus on innovation rather than firefighting.
Real Stories, Real Impact
The numbers tell part of the story, but specific incidents highlight the transformative impact of AI-powered incident response:
The Diwali That Wasn’t Disrupted
Last Diwali, while our competitors struggled with performance issues during peak shopping hours, our systems remained stable despite handling record transaction volumes. The AI had previously identified capacity constraints in our payment processing service and recommended specific scaling adjustments based on projected traffic patterns.
“Our biggest competitor had a four-hour outage during the shopping peak,” one of our sales directors reported. “We gained several major customers directly as a result of our platform’s stability during that critical period.”
The Regulatory Compliance Save
During a routine update to our financial services platform, a subtle configuration change inadvertently affected our audit logging mechanisms – something that could have created serious regulatory compliance issues.
Before any compliance reports were affected, our AI system detected the anomaly in logging patterns and automatically reverted the problematic configuration. The potential compliance issue was resolved before it impacted any regulatory requirements or client operations.
Our compliance officer’s reaction? “This system just saved us from potential regulatory penalties that could have run into crores.”
The Remote Work Reality
When the pandemic forced our sudden shift to remote work, our infrastructure faced unprecedented strains. Our VPN and collaboration tools experienced usage patterns entirely outside historical norms.
Our AI incident system quickly adapted its baselines to the new normal, distinguishing between the expected changes in usage patterns and genuine performance issues. This allowed us to maintain stability despite the dramatic shift in working patterns.
“Other companies struggled for weeks to adapt their monitoring to remote work patterns,” noted our Head of Infrastructure. “Our AI adjusted its baselines automatically within days.”
The Road Ahead: From Incident Response to Self-Healing Systems
Our AI incident response journey continues to evolve. We’re now moving toward truly self-healing systems that can not only detect and diagnose issues but increasingly prevent and resolve them automatically.
Imagine IT systems that:
- Predict capacity needs and scale automatically based on business patterns
- Detect code defects before they reach production
- Automatically optimize performance based on changing conditions
- Learn from near-misses to prevent future incidents
This isn’t science fiction – it’s the practical reality of AIOps (Artificial Intelligence for IT Operations) that forward-thinking companies are implementing today.
How ValueDX Can Help Transform Your Incident Response
At ValueDX, we’ve learned through experience that the journey to AI-powered incident response requires more than just technology. It demands a thoughtful approach that combines the right tools with process changes and cultural shifts.
Our team has guided numerous Indian enterprises across banking, e-commerce, healthcare, and manufacturing through this transformation. We understand the unique challenges of implementing these solutions in the Indian context – from infrastructure considerations to compliance requirements specific to our regulatory environment.
Whether you’re experiencing the pain of frequent outages or simply want to elevate your operational excellence, we’d be honored to share our expertise and help you navigate your journey to AI-powered incident response.
The question isn’t whether you can afford to implement AI incident response. In today’s digital-first world, the real question is: can you afford not to?
Connect with our team today to explore how we can help you reduce downtime, boost productivity, and transform incident response from a reactive necessity to a strategic advantage.
Author: Gajanan Kulkarni